Generative artificial intelligence (AI) is revolutionizing the drug discovery process, offering unprecedented speed and efficiency in developing new treatments, as highlighted in a study published in Nature Machine Intelligence. The research, conducted by a team from Stanford University and the University of Cambridge, demonstrates how AI can design novel drug candidates by predicting molecular structures with remarkable accuracy. This breakthrough, while promising, also raises questions about resource demands, ethical considerations, and the digital divide, reflecting broader trends in AI-driven creative tools and AI privacy debates.
The study, titled “Generative AI for Drug Discovery: A New Paradigm,” explores how AI models can generate and optimize drug candidates by simulating chemical interactions at a molecular level. Nature Machine Intelligence reports that these models can predict the efficacy and safety of potential drugs, reducing the time and cost associated with traditional drug development. Phys.org notes that this technology could accelerate the discovery of treatments for diseases like cancer, Alzheimer’s, and rare genetic disorders, aligning with advancements in AI communication tools that enhance data analysis capabilities.
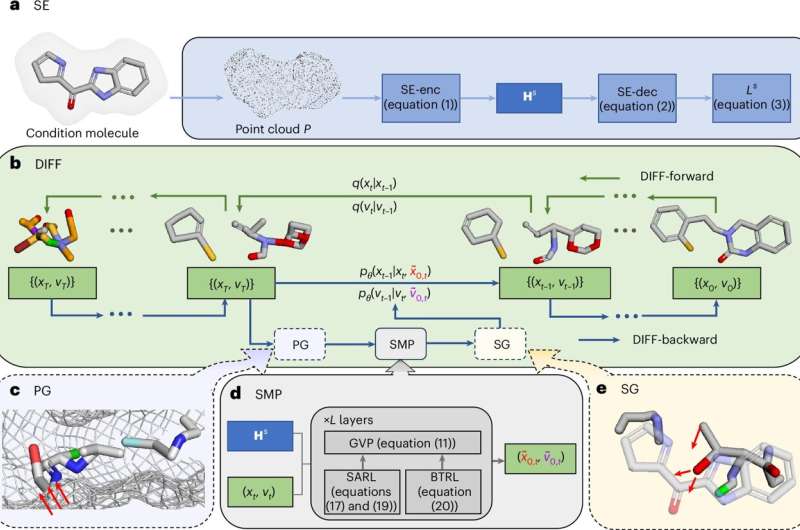
Generative AI works by training on vast datasets of existing drugs and their properties, then generating new molecules that could potentially treat specific conditions. The Stanford-Cambridge team used deep learning algorithms to create a model that can design drugs with desired properties, such as targeting specific proteins or minimizing side effects. This approach has already shown promise in identifying candidates for clinical trials, a process that traditionally takes years. The study’s findings suggest that AI could shorten this timeline to months, a significant leap forward in medical research.
However, the integration of generative AI into drug discovery is not without challenges. The technology requires substantial computational resources, which could limit its accessibility to smaller research institutions, a concern often raised in AI accessibility efforts. Additionally, the ethical implications of AI-generated drugs must be considered, particularly regarding patent rights and the potential for bias in the training data. This aligns with discussions in cybersecurity discussions, where the responsible use of AI is crucial.
The digital divide also poses a significant hurdle. While large pharmaceutical companies may readily adopt this technology, smaller firms and developing countries may struggle to access the necessary infrastructure, potentially exacerbating disparities in healthcare innovation. This challenge is often explored in AI-driven public safety tools, where the balance between innovation and accessibility is critical. Furthermore, the reliance on AI could lead to a concentration of power in the hands of a few large corporations, raising questions about the democratization of medical research.
The transformative potential of generative AI in drug discovery is immense. It offers the promise of faster, more personalized treatments, potentially saving millions of lives. However, its success will depend on addressing these challenges and ensuring that the benefits are widely shared. The technology’s ability to simulate complex biological processes could lead to breakthroughs in treating previously intractable diseases, but it also requires careful oversight to prevent misuse or unintended consequences.
Moreover, the rapid advancement of generative AI in drug discovery could reshape the pharmaceutical industry. Traditional methods, which rely heavily on trial and error, may become obsolete as AI-driven approaches dominate. This shift could lead to a more efficient and cost-effective drug development process, but it also risks displacing jobs in the industry. The balance between technological progress and human expertise will be crucial, a theme often seen in AI language tool debates about the role of AI in professional fields.
The ethical considerations are particularly important. As AI generates drug candidates, questions about ownership and intellectual property arise. Who owns the rights to an AI-designed drug? How should profits be distributed? These issues are complex and will require new legal frameworks to address. Additionally, the potential for bias in AI models, if not properly managed, could lead to drugs that are less effective for certain populations, a concern often raised in AI privacy debates.
In conclusion, generative AI’s role in transforming drug discovery is both exciting and challenging. It offers the potential to revolutionize healthcare, but it also demands careful consideration of its ethical, social, and technical implications. As this technology continues to evolve, the balance between innovation and responsibility will be key. What do you think about generative AI’s impact on drug discovery—will it lead to a new era of medicine, or does it pose significant risks? Share your thoughts in the comments—we’d love to hear your perspective on this transformative technology.